Control
Digital twins monitor changes in the real world, sending data to virtual models to generate insights. To move from these insights to outcomes, interventions from digital twins must be sent to the physical world. Historically, this relationship involved interactions between humans and machines, where humans were required to operate, train or improve systems. This human-in-the-loop approach is common across many industries, and indeed day-to-day life. Given the goal of ‘bake a cake’, a human can translate this end goal to a method, and would then send instructions to machines, like mixers and ovens.
In more complex areas with more complex goals, humans can require vast amounts of knowledge and experience. What if we could transfer this knowledge to machines, revolutionising human-in-the-loop?
Leveraging ontologies allows CMCL to represent data and information, building a consistent information model. Delivering information that is interpretable, interconnected and meaningful allows machines to understand human-generated data, unlocking new possibilities. Given enough data (and machine-controllable hardware) this allows machines to transition directly from a goal to execution. These goals could be ‘reduce energy usage in this neighbourhood’, or ‘make this infrastructure network more resilient’.
We have already delivered a case study, where when given the goal ‘optimise this aldol condensation reaction’, two labs in different continents autonomously generated a range of optimal experimental conditions. In addition, when one of the labs experienced a hardware failure, software agents notified the lab manager and shifted the workload to the other lab.
Applications
Optimisation
Allowing computational agents to optimise processes can pay dividends in cost and time savings.
Automation
Freeing humans from repetitive tasks and letting machines automate processes improves speed and repeatability.
Scale-up
Leveraging automated equipment allows for near limitless scale-up across experiments and other slow processes.
Case Study: Self-Driving Labs
This work is an example of a digital twin leveraging domain knowledge, ontologies and dynamic knowledge graphs to control physical hardware, unlocking innovative new possibilities.
Self-Driving Laboratories are part of a a rapidly growing field that could potentially unlock rapid advances in material design, drug discovery and more. By combining artificial intelligence with automated robotic platforms, experiments can be planned and carried out with far greater speed than before, releasing highly-skilled researchers from repetitive tasks.
However, challenges with scaling the technology have so far hindered progress and limited potential. The immediate need for vaccines during the Covid pandemic highlighted the need for research to be more agile than ever.
The World Avatarâ„¢ and the Derived Information Framework are ideally placed to tackle this challenge, and were applied to connect physical labs in Cambridge and Singapore. The connected laboratories were able to collaborate and rapidly move towards a combined set of results.
More information about this work can be found in this paper.
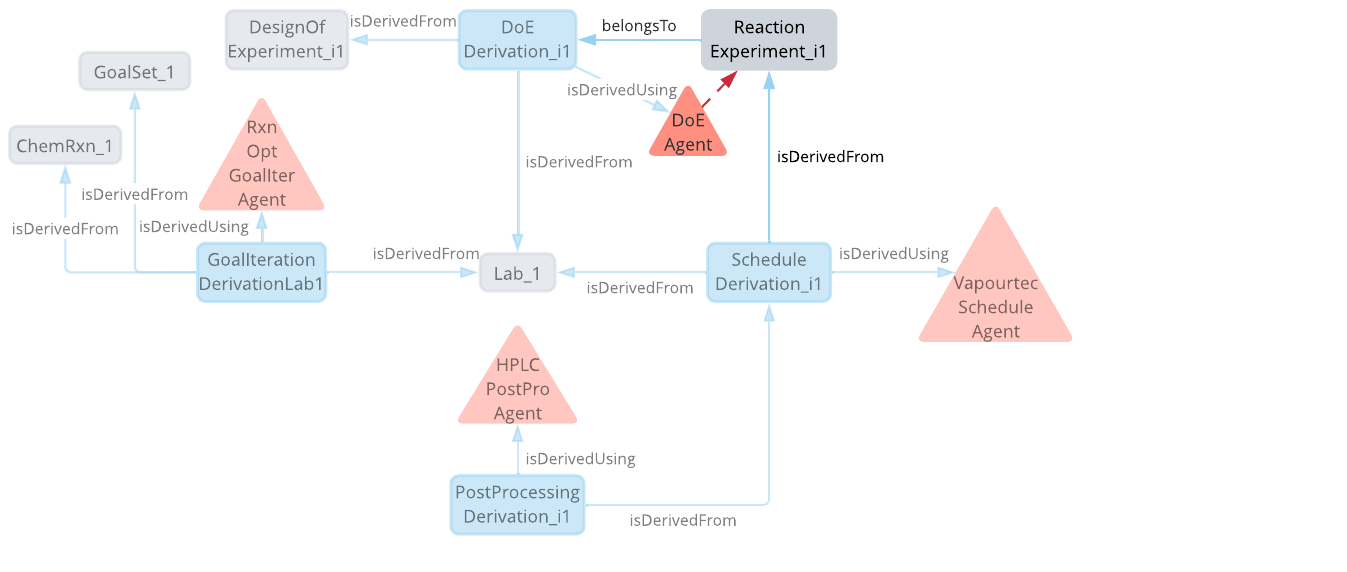
Agents within the framework cascaded information across a dynamic knowledge graph.
Results informed a dynamic Design of Experiment agent, which then continuously suggested new experimental conditions to be executed across both labs.
As experiments were carried out and results gathered, a Design of Experiment (DoE) agent autonomously identified new experimental inputs and passed those parameters to the labs. These points can be seen in the far right chart.
In this case study, the linked labs were able to rapidly (<3 days) generate a Pareto front displaying cost-yield optimisation, a common goal across industry.